Fairness is one of the five principles set out in the government's Artificial Intelligence (AI) white paper, yet it is clear that more must be done to address the bias present in AI systems.
Around 15% of all businesses have incorporated at least one form of artificial intelligent technology in their business according to the key findings of Capital Economics 'AI Activity in UK Businesses' report in 2022. Whilst it is clear that AI has multiple benefits to businesses, including reducing operational costs, improving customer experience, increasing efficiency and potentially growing revenue, the evidence of bias in AI output is and should be a concern to business leaders. In particular when service providers use AI, this can have significant impact on its users and, as such, bias needs to be addressed to ensure AI systems are not unlawful.
AI Output
Before discussing the latest AI guidance on fairness, it is important to understand why the current deployment and use of AI can participate in spreading unfair and negative bias information about members of underrepresented groups (URGs).
Presently the most common AI solutions deployed within companies include data management solutions and natural language processing and generation (NLG) such as Alexa, Siri and ChatGPT. However, one of the key issues stemming from the use of AI software is that its accuracy is dependent on the data it is trained on. AI technologies do not have fundamental beliefs and generate new information simply by mimicking and reproducing existing information, without taking into account characteristics of the end user such as sex, age, or race. In fact, ChatGPT (which is an AI language model) stated when asked to 'write a blog post about how ChatGPT is sometimes biased':
"…ChatGPT has access to an immense amount of data and knowledge, making it a valuable tool for individuals seeking information or advice on various topics. However, like all AI models, ChatGPT is not perfect and can sometimes exhibit bias in its responses.
Bias can manifest itself in several ways. For instance, ChatGPT may be trained on a particular dataset that reflects certain cultural or societal norms, resulting in it producing responses that may be insensitive or even discriminatory towards certain groups of people. Additionally, ChatGPT may unintentionally perpetuate certain stereotypes or reinforce existing biases that are prevalent in society."
As a result, responses, articles, or essays produced, can present information that is racially charged, politically incorrect and offensive to URG users. For example, when ChatGPT was recently asked to create a formula to assess whether someone would be a good scientist, based on the description of their race and gender, it responded by saying a good scientist is white and male. As such the risks of AI outputs engaging in incorrect stereotypes, harmful dialogue and, as by-product potentially unfair treatment, are imminent.
Next steps towards a fairer approach
In response to the growing concerns around fairness, the UK's Department for Science, Innovation, and Technology (the DSIT) has continued to work towards creating the right environment to harness the benefits of AI, including getting regulation right and addressing risks posed by AI, such as ensuring that fairness is a key regulatory guiding factor when deploying and using AI.
A key factor to mitigate the fairness risk posed, is that the detection of bias requires AI technology to have access to demographic data such as the sex, age, and race of users of the AI. Developers of AI have often struggled to obtain this for practical, legal, and ethical reasons, particularly in light of data protection laws. For this reason, on 14 June 2023, the Centre for Data Ethics and Innovation (the CDEI) and the DSIT published a report exploring the possibilities of enabling responsible access to demographic data to mitigate the risks of AI systems fairer (the "Report").
The Report sets out two ways which circumvent the need to directly collect demographic data which involve AI developers engaging with and using:
- Data intermediaries – covering a range of different activities and models that can support the access to, and facilitate the sharing of, data. The use of social media platforms as identity intermediaries to sign onto websites would be an example of a data intermediatory; and
- Proxies – enable demographic data to be inferred in one data set based on proxy data already held. For example, using postcode data to infer ethnicity to avoid the need to access the individual's privately held data.
The above, whilst promising, is likely to be limited in progress at present. For example, the Report notes that there are currently no intermediaries offering this type of service in the UK, but notes that this gap may be due to the UK being a "first mover in this complex area". Further, in respect of proxy tools, significant care is needed for compliance with data protection law as inferred demographic data is likely to fall under personal or special categories of data under the GDPR. In the absence of a cautious and diligent approach there could be damaging inaccuracies or risks to users' privacy and autonomy.
The CDEI has also published a blog on its fairness innovation challenge, drawing on the Government's March 2023 whitepaper on the UK's pro-innovation approach to AI regulation. This blog reinforces the importance of fairness in AI systems and discussed plans to run a fairness innovation challenge to assist with the development of holistic solutions to address bias across the AI lifecycle.
Key AI considerations for your business
Taking into account the recent guidance on AI usage and the emphasis on fairness, businesses wishing to incorporate AI into their internal systems or advocate the use of external AI should consider the following:
- AI Policy – ensure the agreement to and creation of an AI policy in your business which outlines the benefits and seeks to minimise potential risks and discriminatory outcomes (for example that may contravene the Equality Act 2010, Human Rights Act 1998, or any similar legislation), making sure output is moderated and checked and that any policy documents are kept up to date in line with any changes in guidance;
- Due Diligence of AI Suppliers – question your suppliers of AI technology by producing a due diligence questionnaire before onboarding; have they taken steps to ensure fairness in the development of their own AI; can they evidence the use of data intermediates, proxies and/or other fairness solutions; and check the quality of the AI's training data;
- Test Models and Pilots – before deployment of any AI, in addition to undertaking the necessary diligence checks, run the AI system for a test screening specifically for bias and fairness before subscribing or implementing systems;
- Ongoing Monitoring – businesses should have an internal process to help identify any bias or discriminatory outcomes generated by their existing AI technology and report to the relevant AI supplier, to help rectify any systematic errors if possible and/or limit the use of the AI system in certain respects where the outcomes are harmful; and
- Bias Consciousness and Training – when using an AI system, it is important that you stay aware of the possible unfair outcomes, for example, reviewing all articles and responses from NLGs such as ChatGPT and providing training / guidance for employees using AI to ensure a company-wide approach is adopted.
Conclusion
Whilst there is a concern around the fairness of AI technology outcomes, it is clear that one of the predominant reasons for biased output stems from the AI technology being trained on either incomplete or publicly available biased data.
Bias is as much a human problem as it is a technological one. In order to reduce the risks of AI producing subjective results, AI developers should seek to use data intermediators and proxy tools to help gain access to demographic data and develop AI systems to generate fairer outcomes and responses. It is important to ensure an agreed internal policy is in place, due diligence checks on suppliers are undertaken, AI systems are tested and monitored, and users stay conscious of AI bias when reviewing and utilising any data produced by AI technology.
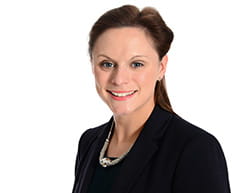
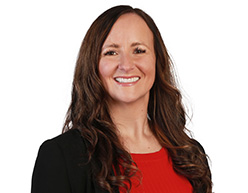